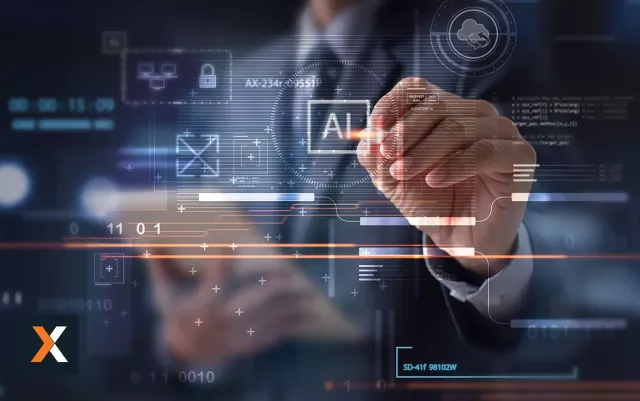
For a moment, let’s leave the safe blanket of the ‘pipeline envelope’. This warm and cozy place is when we have a lead, assign it a close probability, and can comfortably forecast a sale. But what about those deals that have not entered this envelope? For these, we need to use models that usually rely on historical data. Statisticians pull out every regression and moving average model in their toolkit to find the methodology that creates the best forecast. But what if there is no historical sales data or historical sales data is not an accurate predictor of the future?
Crickets…
I once saw a CFO forecast using an actual dartboard with random forecasts stapled to it that was hung in his office. When I noted to him that this would not instill much confidence in the accuracy of his numbers, he tactfully noted that he had a better track record than the past three people who held his role – each for a very short time. I would have paid a small fortune to own that dartboard.
What are we to do if we want long-term forecasts (beyond the pipeline envelope) but do not have any historical sales data? There are options.
Comparable Capacity
If you have access to market surveys that show the productivity of sales representatives in the industry, then you should expect that your team will produce a similar amount (per representative). A few things need to happen for this methodology to work, or be practical. This methodology assumes that the cost of producing a dollar in sales is consistent in an industry. The underlying assumption is that a rep has an assumed productivity rate or ROI within an industry (which kind of makes sense). PE firms think this way as well - a rep should produce 5X their total pay, and this changes by industry and company size. Therefore, quotas and pay compared to the market can be used to determine the ROI compared to the market.
This methodology assumes that the staffing plan is fixed by investment limitations (‘we can only get this much money for a team, so what will they produce?’). This is a bit backward from the best practice model where you hire enough reps based on their assumed capacity to meet a sales forecast, but when you are missing the denominator of an equation you do what you can.
Lagging Indicator / Vector Autoregression
I have seen this methodology used in many industries, most recently in medical devices. I worked with a company that produced a device to remove cancerous skin cells. It was impossible to use historical data to know how much they would sell because cancer rates and device demand fluctuated according to how much sun people were exposed to (region) and age (demographics). However, the company knew that a surefire way to forecast future demand was to look at the number of cancer screenings that occurred three months ago. If there was an uptick in a region in screenings, three months later device demand would increase among doctors. For each 150 screenings, there would be one device demanded in an area in three months. The model required looking at market data and identifying the amount of lag required to produce a high correlation with an increase in demand (lagging the data by 4 months produced a very low correlation, but lagging it by 3 months had a very high correlation).
There is a fancy regression formula for two-time series of data to test correlation that requires lagging the data of one-time series to increase the correlation (minimize the errors). This is called a vector autoregression – feel free to replace “lag the data” with “vector autoregression” when you want to impress people at your next work party.
Bass Diffusion
This methodology was all the craze many years ago at high-tech companies and is primarily used to forecast new product sales — but it does require historical sales data from other new product launches. Someone noticed that when a new innovative product was launched (e.g., the first cell phone, a flying car), there was a period when people would adopt the new technology. It takes time for a product to penetrate the market, and if the market potential is known then a forecast can be made. This model makes a good amount of assumptions and requires some pretty accurate inputs that are usually not realistic (speed of customer adoption, probability of market imitation, rate of consumption of market potential), but if the data exists and you have a very eager statistician working for you, then it can be done. There are tables available that list historical market values, and if your company has a track record of launching innovative products then the inputs into the model should be there.
Interestingly, this methodology produces a highly realistic sales lifecycle where there is a steep curve upward as the market adopts the technology. There is then a plateau as imitators enter the market and the market has adopted the product, followed by a steady decline in demand due to saturation. Truly innovative companies will time launches based on the lifecycle curve predicted by the Bass Diffusion model.
Test Markets
This is the option that most companies follow – when data doesn’t exist, create some. The idea is to target a small but representative sample where market potential can be established. The results are assumed to be scalable, so a larger forecast can be made. The obvious downside to the approach is that it takes much longer to arrive at a number. Additionally, finding a test market is not that easy, and it often takes several test markets to find one that is representative of the larger market. This adds even more time to arriving at an accurate forecast. For example, one test market may require more advertising, or it may have a quicker adoption rate due to higher technical skills and thus is not representative enough to build an aggregate forecast.
Surveys
There are many types of surveys, and I could not possibly list them all. There are two general types that I have seen used to derive a forecast, and I would love to say that they are awful, but remember the dartboard story? The first type is the sentiment survey where a large group is polled. This large group can be internal (e.g., sellers), or external (e.g., potential buyers) about their beliefs on potential sales. I am sure everyone has seen these surveys pop up online (usually right before a video plays) – ‘are you intending to purchase such-and-such products next year?’ This is a sentiment survey and can be used to forecast sales. These surveys usually have a short shelf-life and need to be done with a good amount of frequency.
The second type is the Delphi survey. This involves a qualitative survey of a large group of industry experts on what they believe the market will produce and it is up to the company to determine their market share. This is a very popular methodology with academics – probably because they are usually the ones being surveyed. However, it is not hard to imagine a company looking at a well-known group of experts (e.g., an investment bank), using their projection for the total sales in a specific market next year, and then applying their historical market share to this number to derive a forecast.
Some key insights can be gained from using these methods even if you have historical data to leverage for a sales forecast. For example, most sales forecasts tend to be moving averages or regression lines, so if your company’s sales increased in the past few years the forecast will most likely show an increase for next year. The lack of knowledge that a survey would provide (or a Bass Diffusion model would predict, or a test market may point towards, or a lagging indicator may forecast) may cause you to miss a leveling off in demand. Each of these methods is another piece that can be added to a larger quilt that can keep you more secure and warm beyond the cozy blanket of the pipeline envelope.
Happy hunting.